Developing large-scale datasets has been critical in computer vision and natural language processing. These datasets, rich in visual and textual information, are fundamental to developing algorithms capable of understanding and interpreting images. They serve as the backbone for enhancing machine learning models, particularly those tasked with deciphering the complex interplay between visual elements in images and their corresponding textual descriptions.
A significant challenge in this field is the need for large-scale, accurately annotated datasets. These are essential for training models but are often not publicly accessible, limiting the scope of research and development. The ImageNet and OpenImages datasets, containing human-annotated images, are incredibly valuable resources for visual tasks. However, when it comes to functions that merge vision and language, datasets such as CLIP and ALIGN, despite their richness, are not widely accessible, posing a bottleneck in the advancement of this domain. Floor Trusses

The “Let’s Go Shopping” (LGS) dataset is a groundbreaking resource that fills an important gap. Developed to enrich visual concept understanding, LGS is a comprehensive dataset comprising 15 million image-description pairs culled from approximately 10,000 e-commerce websites. Its primary focus is to enhance the capabilities of computer vision and natural language processing models, specifically in e-commerce. This dataset is a notable departure from the traditional datasets, focusing more on objects in the foreground with simpler backgrounds, a characteristic feature of e-commerce images.
The methodology behind LGS, curated by researchers from the University of California, Berkeley, ScaleAI, and New York University, is as meticulous as innovative. The dataset’s images predominantly feature products against clear backgrounds, enhancing the models’ ability to focus on the object of interest. LGS contrasts with the typical datasets where the subject often blends into a complex background. The collection process involved a semi-automated pipeline that efficiently gathered product titles, descriptions, and corresponding images while ensuring high-quality data. The data spans a wide range of products, from clothing to electronics, providing diverse visual and textual information.
The performance of the LGS dataset in various applications highlights its utility. Models trained on LGS have improved performance in tasks like image classification, reconstruction, captioning, and generation, especially in e-commerce. The dataset’s unique distribution and high-quality image-caption pairs significantly enhance the model’s understanding of e-commerce-specific visual concepts. This aspect of LGS is particularly beneficial for applications where understanding the subtleties of product images and descriptions is crucial.
The introduction of the LGS dataset is a leap forward in visual concept understanding, particularly in e-commerce. It fills a critical void in the availability of large-scale, high-quality datasets for vision-language tasks. The LGS dataset enriches the resources available to researchers and developers. It opens new avenues for innovative research and application development in the intersecting fields of computer vision and natural language processing. With its distinct focus on e-commerce imagery and descriptions, LGS is a testament to the evolving landscape of machine learning datasets, paving the way for more specialized and accurate models in this ever-expanding domain.
Check out the Paper. All credit for this research goes to the researchers of this project. Also, don’t forget to follow us on Twitter. Join our 36k+ ML SubReddit, 41k+ Facebook Community, Discord Channel, and LinkedIn Group.
If you like our work, you will love our newsletter..
Don’t Forget to join our Telegram Channel
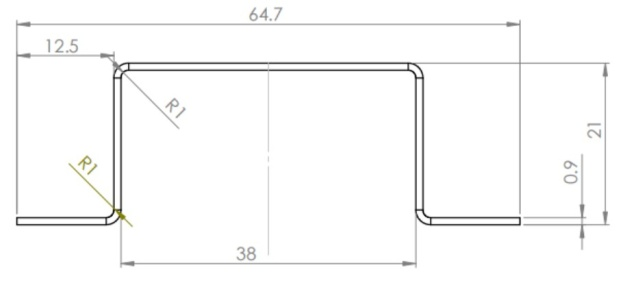
Lightweight Steel Frame Building Muhammad Athar Ganaie, a consulting intern at MarktechPost, is a proponet of Efficient Deep Learning, with a focus on Sparse Training. Pursuing an M.Sc. in Electrical Engineering, specializing in Software Engineering, he blends advanced technical knowledge with practical applications. His current endeavor is his thesis on "Improving Efficiency in Deep Reinforcement Learning," showcasing his commitment to enhancing AI's capabilities. Athar's work stands at the intersection "Sparse Training in DNN's" and "Deep Reinforcemnt Learning".